Know the real-world examples, key methodologies, and the power of an iterative mindset in shaping the future of ethical artificial intelligence.
Background
To start, Daniel, could you please provide a brief introduction to yourself and your work at Kognic?
I’m an experienced machine-learning expert and passionate about making AI useful for safety critical applications. As CEO and Co-Founder of Kognic, I lead a team of data scientists, developers and industry experts. The Kognic Platform empowers industries from autonomous vehicles to robotics – Embodied AI as it is called – to accelerate their AI product development and ensure AI systems are trusted and safe.
Prior to founding Kognic, I worked as a Team Lead for Collection & Analysis at Recorded Future, gaining extensive experience in delivering machine learning solutions at a global scale and I’m also a visiting scholar at both MIT and UC Berkeley.
Overview
Can you give our audience an overview of what AI alignment is and why it’s important in the context of artificial intelligence?
AI alignment is a new scope of work that aims to ensure that AI systems achieve their desired outcomes and work properly for humans. It aims to create a set of rules to which an AI-based system can refer when making decisions and to align those decisions with human preferences.
Imagine playing darts, or any game for that matter, but not agreeing on what the board looks like or what you get points for? If the product developer of an AI system cannot express consistent and clear expectations through feedback, the system won’t know what to learn. Alignment is about agreeing on those expectations.
AI Alignment and Embodied AI
How does ensuring AI alignment contribute to the safe and ethical development of Embodied AI?
A significant amount of the conversation around AI alignment has been on its ability to mitigate the development of a ‘God-like’ super powered AI that would no longer work for humans and potentially pose an existential threat. Whilst undoubtedly an important issue, such focus on AI doomsday predictions doesn’t reflect the likelihood of such an eventuality, especially in the short or medium term.
However, we have already seen how the misalignment of expectations between humans and AI systems has caused issues over the past year, from LLMs such as ChatGPT generating false references and citations to its ability to generate huge amounts of misinformation. As Embodied AI becomes more common – where AI is embedded in physical devices, such as Autonomous Vehicles – AI Alignment will become even more integral to ensure the safe and ethical development of AI systems over the coming years.
Could you share any real-world examples or scenarios where AI alignment played a critical role in decision-making or Embodied AI system behaviour?
One great example within the automotive industry and the development of autonomous vehicles, starts with a simple question: ‘what is a road?’
The answer can actually vary significantly, depending on where you are in the world, the topography of the area you are in and what kind of driving habits you lean towards. For these factors and much more, aligning and agreeing on what is a road is far easier said than done.
So then, how can an AI product or autonomous vehicle make not only the correct decision but one that aligns with human expectations? To solve this, our platform allows for human feedback to be efficiently captured and used to train the dataset used by the AI model.
Doing so is no easy task, there’s huge amounts of complex data an autonomous vehicle is dealing with, from multi-sensor inputs from a camera, LiDAR, and radar data in large-scale sequences, highlighting not only the importance of alignment but the challenge it poses when dealing with data.
Teaching machines
Teaching machines to align with human values and intentions is known to be a complex task. What are some of the key techniques or methodologies you employ at Kognic to tackle this challenge?
Two key areas of focus for us are machine accelerated human feedback and the refinement and fine-tuning of data sets.
First, without human feedback we cannot align AI systems, our dataset management platform and its core annotation engine make it easy and fast for users to express opinions about this data while also enabling easy definition of expectations.
The second key challenge is making sense of the vast swathes of data we require to train AI systems. Our dataset refinement tools help AI product teams to surface both frequent and rare things in their datasets. The best way to make rapid progress in steering an AI product is to focus on that which impacts model performance. In fact, most teams find tons of frames in their dataset that they hadn’t expected with objects they don’t need to worry about – blurry images at distances that do not impact the model. Fine-tuning is essential to gaining leverage on model performance.
In your opinion, what role does reinforcement learning play in training AI systems to align with human goals, and what are the associated difficulties?
Reinforcement Learning from Human Feedback (RLHF) uses methods to directly optimise a language model with human feedback. While RLHF has enabled large language models (LLMs) to help align previously trained output, those models work from a general corpus of text. In Embodied AI, such as Autonomous Driving, the dataset is far more complex. This includes video, camera, radar, LiDAR plots of varying sequence and time, other vehicle sensor data such as temperature, motion, pressure…. Human feedback in this context can be reinforced, but automation will only get you so far.
The iterative mindset
Daniel, you have mentioned the importance of an iterative mindset in AI. Could you explain what you mean by this and why it’s crucial to the development of AI systems?
We believe that good things come to those who iterate. We live in a fast changing world and data sets used to train and align AI systems have to reflect that. AI systems and products are generating and collecting huge amounts of data and given the reality that data doesn’t sleep, there is a need for both flexibility and scale when optimising. Our tools are designed with this in mind, making it easy to change decisions based on new learnings and to do so at a lower cost.
Many businesses aren’t comfortable working in this manner. The automotive sector for instance has traditionally operated on a waterfall methodology, but it is absolutely vital we see a mindset shift if we are to successfully align AI systems with human expectations.
Can you share any specific strategies or best practices for implementing an iterative mindset in AI alignment projects?
One strategy is to remap software organisations inside enterprises to think about “programming with data” versus “programming with code”. For this, the skill sets of product developers, engineers and other technical staff need to be adept and comfortable with exploring, shaping and explaining their datasets. Stop trying to address machine learning as a finite process, but rather an ongoing cycle of annotation, insights and refinement against performance criteria.,
Final thoughts
To wrap up, what advice would you give to organisations or researchers who are actively working on AI alignment and ethics in artificial intelligence? What steps can they take to make progress in this field?
Our team at Kognic is bullish on AI and the promise that it can improve our world. For this, the biggest advantage to exploit is a mindset that consistently challenges the immediate way of working – particularly when results from an AI product are not what you expect. We are telling the “machine” what to aspire to, what to aim for… And that job doesn’t end. Keep at it and improvements will cascade into more efficient and safer AI experiences.
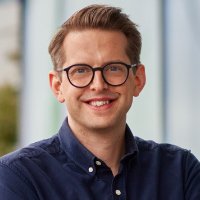
Daniel Langkilde
CEO and Co-founder of Kognic
Daniel Langkilde, CEO and co-founder of Kognic, is an experienced machine-learning expert and leads a team of data scientists, developers and industry experts that provide the leading data platform that empowers the global automotive industry’s Automated Driver Assist and Autonomous Driver systems known as ADAS/AD. Daniel is passionate about the challenge of making machine-learning useful for safety-critical applications such as autonomous driving.
Prior to founding Kognic, Daniel was Team Lead for Collection & Analysis at Recorded Future, the world’s largest intelligence company maintaining the widest holdings of interlinked threat data sets. At Recorded Future, Daniel got extensive experience in delivering machine learning solutions at global scale.
Daniel holds a M.Sc. in Engineering Mathematics and has been a Visiting Scholar at both MIT and UC Berkeley.