Much of the historical progress has been led by AI-native drug discovery companies that offer software or service to pharma players. And facilitating such services by one-of-a-kind organic chemists having expertise in molecular modeling combined with the knowledge of AI can only bring about disruption. So is this inspiring interview with Hanjo Kim, SVP of Global Strategy, Head of Medicinal Chemistry at Standigm.
He believes that AI has the potential to propagate to other intersections of different expertise and improve the overall productivity in drug discovery. To learn more kindly go through the interview highlights below:
Please brief our audience about yourself and your role as the SVP of Global Strategy, Head of Medicinal Chemistry at Standigm.
I’m an organic chemist by training and education. I have worked for various organizations, a non-profit research organization, a biotech, and a pharmaceutical company, before joining Standigm in 2019. I led the development of Standigm BEST, its chemistry technology, until 2021. From 2022, my role has changed to half research, head of medicinal chemistry, and the other half business development.
Please share your source of inspiration for exploring various facets of technology.
As an organic chemist with molecular modeling expertise, I have spent my entire professional career living on the edge of two totally different disciplines; wet lab and dry lab. As these two have different philosophies, customs, and ways of thinking, my primary job has been as a translator or a facilitator in most cases. Therefore, it was natural for me to join Standigm and deal with combining AI and drug discovery, two different technologies. The questions from experts with diverse backgrounds are a trustworthy source of inspiration.
Kindly brief our audience about Standigm and give us an overview of its standout solutions.
Standigm, which comes from the ‘standard paradigm,’ is a start-up founded in 2015 by three co-founders who are former SAIT (Samsung Advanced Institute of Technology) colleagues. It has over fifty employees, most of whom are R&D scientists with different expertise, such as AI algorithms, software engineering, chemistry, and biology, in its three offices, headquartered in Seoul, South Korea, Standigm UK in Cambridge, UK, and Standigm US in Boston, USA. Standigm focuses on early drug discovery, which can be defined as the combination of novel biology and novel chemistry.
Standigm ASK is a set of processes to identify promising and novel target proteins for a given disease. It uses multi-modal methods, a general knowledge-level approach using knowledge graphs and AI algorithms, various ways to use experimental data (mostly multi-omics data from patient samples) for the specific contexts of biological systems, and many filters. Standigm BEST is a collection of different workflows for hit identification, hit-to-lead, and lead optimization, and their components, generative models, prediction models, and automated learning systems. By combining these two techniques, Standigm could shorten the time to get confirmed lead compounds to an average of seven months.
Being a thought leader and having extensively supported drug discovery since a long time, how important is the role of AI in accelerating drug discovery?
The role of AI in drug discovery differs from older computer-aided drug design technologies in that it can complete a closed-loop system running automatically. In early drug discovery projects, the DMTA (Design-Make-Test-Analyze) cycle should run many times to optimize the molecules. In this cycle, the “design” part requires a high level of creativity and a substantial understanding of the “make” process, so it has been the role of well-trained medicinal chemists. The “analyze” part eventually was to persuade the “designer.” The generative AI models which generate novel chemical structures help the “design” process be scalable, eventually making the whole DMTA cycle faster and easier.
As data price is the most significant drug discovery problem, adopting AI means more DMTA cycles, generating more high-quality data with the same resources.
What is your opinion on integrating technological advancements like AI and ML in the medicinal chemist niche?
Many might be surprised to hear that the first computer-aided drug design technique emerged in 1964. Medicinal chemists are arguably one of the earliest scientists to adopt new technologies. The fundamental problem of AI-assisted chemistry is the lack of high-quality and high-volume data caused by the importance of patents (intellectual property) in the pharmaceutical industry and the expensive experiments required. From this perspective, AI and ML technology should work with automation to produce high-quality and high-volume data at a lower cost.
Medicinal chemists learn a lot from their experience, which cannot be easily transcribed as all the projects have different contexts. AI technology is an excellent way to transcribe medicinal chemists’ experiences in more transparent and applicable language. It helps medicinal chemists understand their biases, validate their SAR analyses more quantitatively, learn new findings, and integrate them with current calibers.
How will this intersection help computational chemists solve the dynamic challenges of the drug discovery sector?
The barrier between computational and experimental chemists can be overcome only by a transparent data-driven decision process. As AI always asks for more high-quality data to improve its models, these two groups of experts should collaborate to create efficient data streams, the benefits of which both will appreciate. Though this cannot solve all challenges of drug discovery, it propagates to other intersections of different expertise to improve overall productivity in drug discovery.
In your opinion, what is the future of deep learning and generative AI in healthcare?
It depends on the nature of the data, especially their price. The expensive data like activities, druggabilities, and toxicities of molecules won’t be sufficient ever, so few-shot learning methods will be improved and used more than ever. Mathematical models are required to supplement AI models developed with small data. Considering the complexity of the biological system, sophisticated integration of AI models of different levels is a future direction in this field.
What advice would you like to give budding entrepreneurs and leaders about investing in AI drug discovery?
I have broadly seen two groups of people accepting AI technologies. One group regards it as a more efficient tool. As AI is another way to do a task better, validation is more accessible, and return is more prominent while its influence can be limited. The other group tries to change their ways of doing complex workflows to create a novel business logic with the help of AI technology. Though validating is more complicated, it can be far more influential. AlphaGo, AlphaFold2, and ChatGPT are good examples of the previous, but I don’t know any later instances. Though there is no right or wrong selection here, one should understand the final goal as it will affect the journey of projects, businesses, companies, and industries.
How do you plan to scale both–Standigm and your growth curve for 2023 and beyond?
One cannot solve all the problems. Meeting good collaborators, partners, and clients is the key to Standigm’s growth in 2023, more than ever. More profound science can result in more unique and attractive capabilities. Standigm is improving its AI models with the data, results, and human expertise, accelerating its growth in this fast-growing market.
Personally, two distinct roles are a big challenge, but it creates many collisions I have never seen before and encourages me to face more creative challenges. As AI models can be improved by smaller and quicker learning cycles, the repetition of small successes and failures will make Standigm and myself more potent.
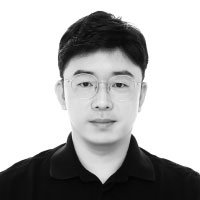
Hanjo Kim
SVP of Global Strategy, Head of Medicinal Chemistry at Standigm
Hanjo Kim is the SVP of Global Strategy, Head of Medicinal Chemistry at Standigm, a workflow AI-driven drug discovery company headquartered in Seoul, South Korea with offices in the U.S. and UK. As SVP of Global Strategy, he leads the company’s business development and public relations teams and as Head of Medicinal Chemistry, he leads the medicinal chemist team and the AI scientist team, focusing on organic synthesis-related AI models. This combination of two groups enables the generative models of Standigm to incorporate the patentability, synthetic accessibility, and activity of molecules in its design process. Prior to joining Standigm, he worked as the Principal Researcher, CJ Healthcare R&D Center, Head, EQUIS & ZAROO R&D Center, and Principal Scientist, EQUIS & ZAROO R&D Center. He holds a Ph.D. in organic chemistry from Yonsei University.